In the few short years since artificial intelligence (AI) arrived on the museum landscape, the technology has wound its way throughout the cultural institution to enhance its offerings as much as its digital infrastructure. Besides audience-facing robots, chatbots, and projects such as The Next Rembrandt and Dali Lives, AI can be found — even when not seen — assisting in digital asset management, visitor tracking, conservation, and data analysis.
A clearer measure of AI’s cultural presence in the findings of EuropeanaTech, a R&D community and offshoot of the European Union’s Europeana initiative that, since 2019, has been interrogating the impact and future role of AI in the sector of GLAMs (galleries, libraries, archives, museums). Its latest report, surveying 56 cultural heritage institutions in 20 countries, found the majority of respondents were AI-literate, with metadata quality, knowledge extraction, and collections management being key areas of interest.
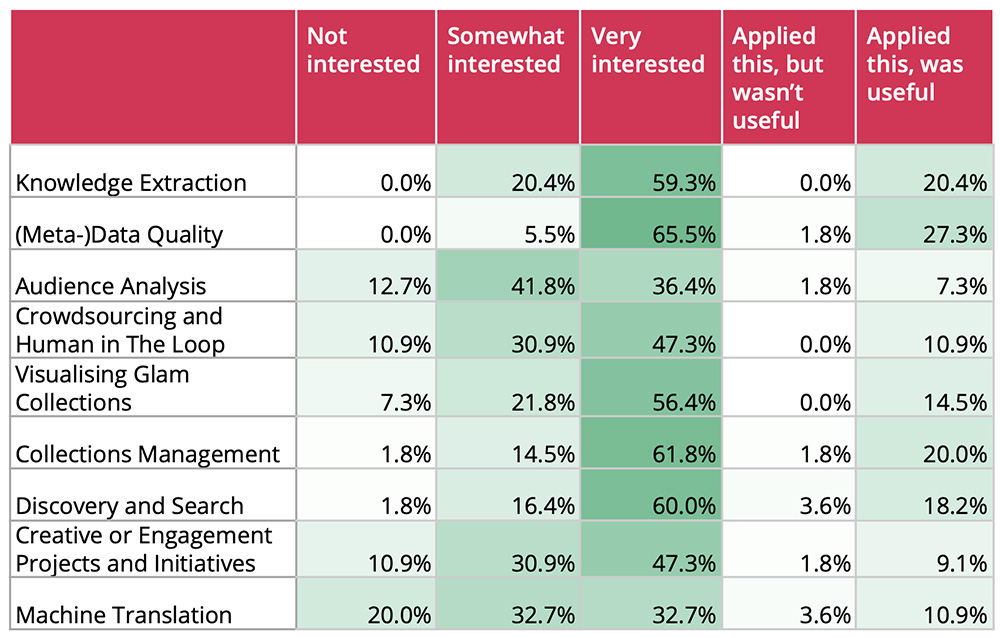
Results to the survey question, “What is your level of expertise or interest in the following AI topics?” recorded the interest of over half of respondents in areas such as metadata quality and collections management. Image: EuropeanaTech
Going deeper, by examining 36 AI use cases, the study determined that GLAMs were most keen on deploying AI around their digitized collections, whether for image classification or object detection to improve metadata. While few of these projects reached production level, almost all achieved tangible results, including trained models, enriched collections descriptions, and enhanced infrastructure.
Still, hurdles persist for AI to get to institution-wide implementation. Through its case studies, EuropeanaTech gathered recurring themes and challenges that faced cultural heritage organizations from the financial cost of hardware and software to the lack of technical know-how. Below are three key concerns accompanied by recommendations from the report.
Tapping and sharing technical expertise
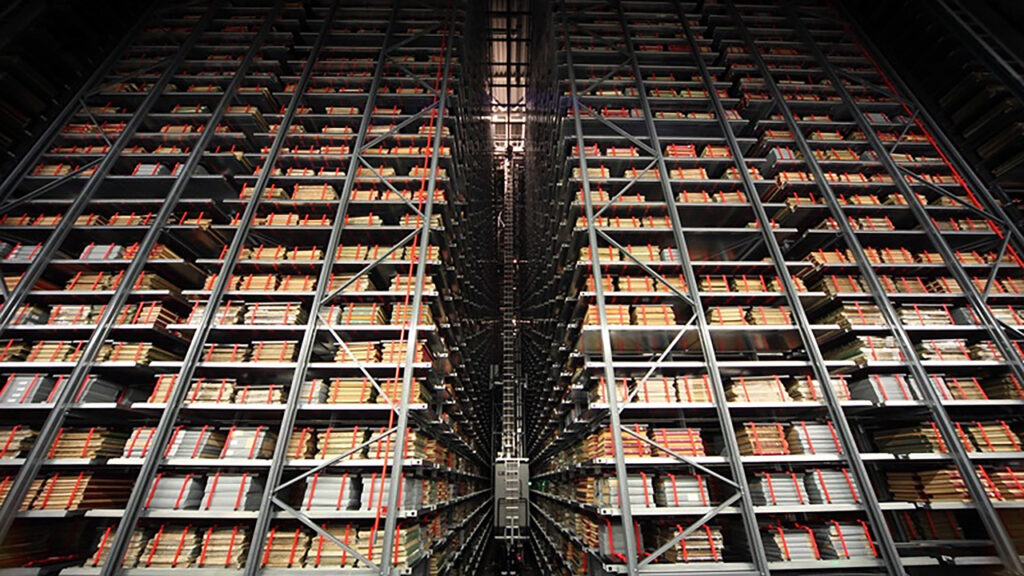
The British Library’s Living With Machines initiative aims to develop new computational techniques for working with historical research questions. Image: Living With Machines
As EuropeanaTech’s report plainly states, “AI in GLAMs is a multidisciplinary endeavor.” AI adoption, then, doesn’t just require cross-departmental buy-in, but a collaborative framework that allows for skill sharing. The British Library, when embarking on its Living with Machines research project to digitize 18th century historical documents, found it helpful to involve both curators and AI specialists from the outset to better pave the way for a shared language. Additionally, the institution, like other GLAMs featured in the report, sought partnerships with commercial and academic groups to make up for its lack of internal AI expertise.
This technical shortfall can be alleviated as well by the use of open source tools and solutions, further customized to address the institution’s needs and data. Transfer learning, in which a machine learning model is retrained for use in different tasks, might also help optimize learning and build-out times, and, according to Barcelona Supercomputing Center’s Albin Larsson, lower the barrier to AI adoption.
Improving data quality
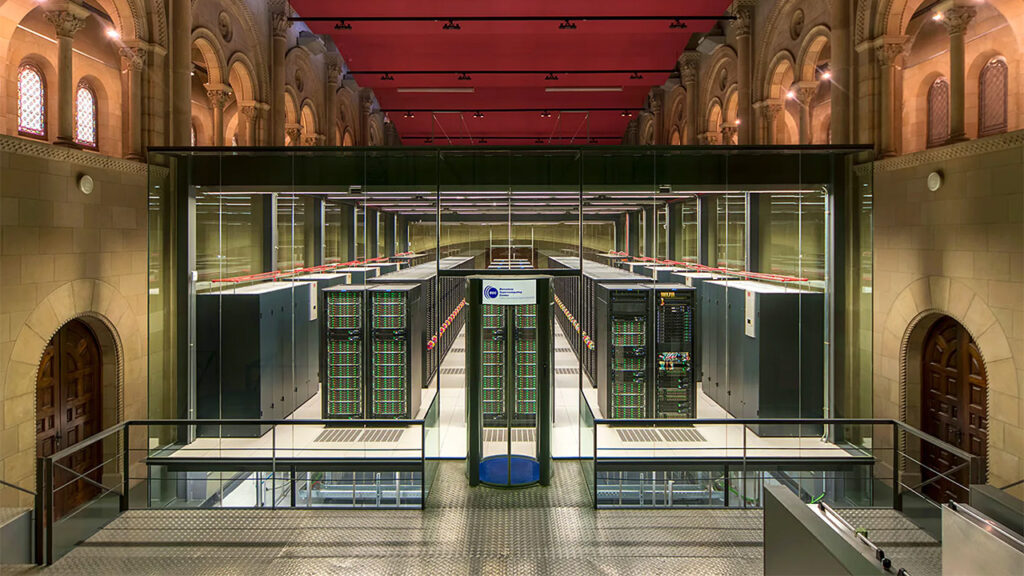
“Knowledge about cultural heritage is very much needed in AI projects,” noted Barcelona Supercomputing Center’s Maria Cristina Marinescu in the report. Image: © courtesy Barcelona Supercomputing Center
Data sits at the heart of any AI endeavor, necessitating the need for quality datasets. But between the institutions interviewed by EuropeanaTech, the valuation of their own datasets varied from “not being reliable yet” to “good enough.” The aforementioned Barcelona Supercomputing Center found it lacked data to generate its machine learning model, while the National Library of France’s datasets remain at an experimental level. Another area of concern lies in the deficit of data with relevant and useful annotations.
To address this, interviewees put forward the option of human computation techniques or, in lay terms, crowdsourcing. Institutions could potentially tap the knowledge of its audience to help with the pre-processing of data or, using a gamified machine learning interface, encourage contributions to accelerate data processing and evaluation.
Adding value to user experience
Demonstrating the value of AI remains the biggest hurdle for most teams and per EuropeanaTech’s study, can be best achieved at scale. As Tim Manders of the Netherlands Institute for Sound and Vision noted of AI, “You only see the advantages if you do it at a big enough scale.” Running AI processing at collection scale, the report suggests, easily highlights its benefits and efficiencies, while enabling data to be presented in a meaningful way. And it’s only with such coherent representation can cultural heritage data be truly significant in generating insights and enriching user experiences.